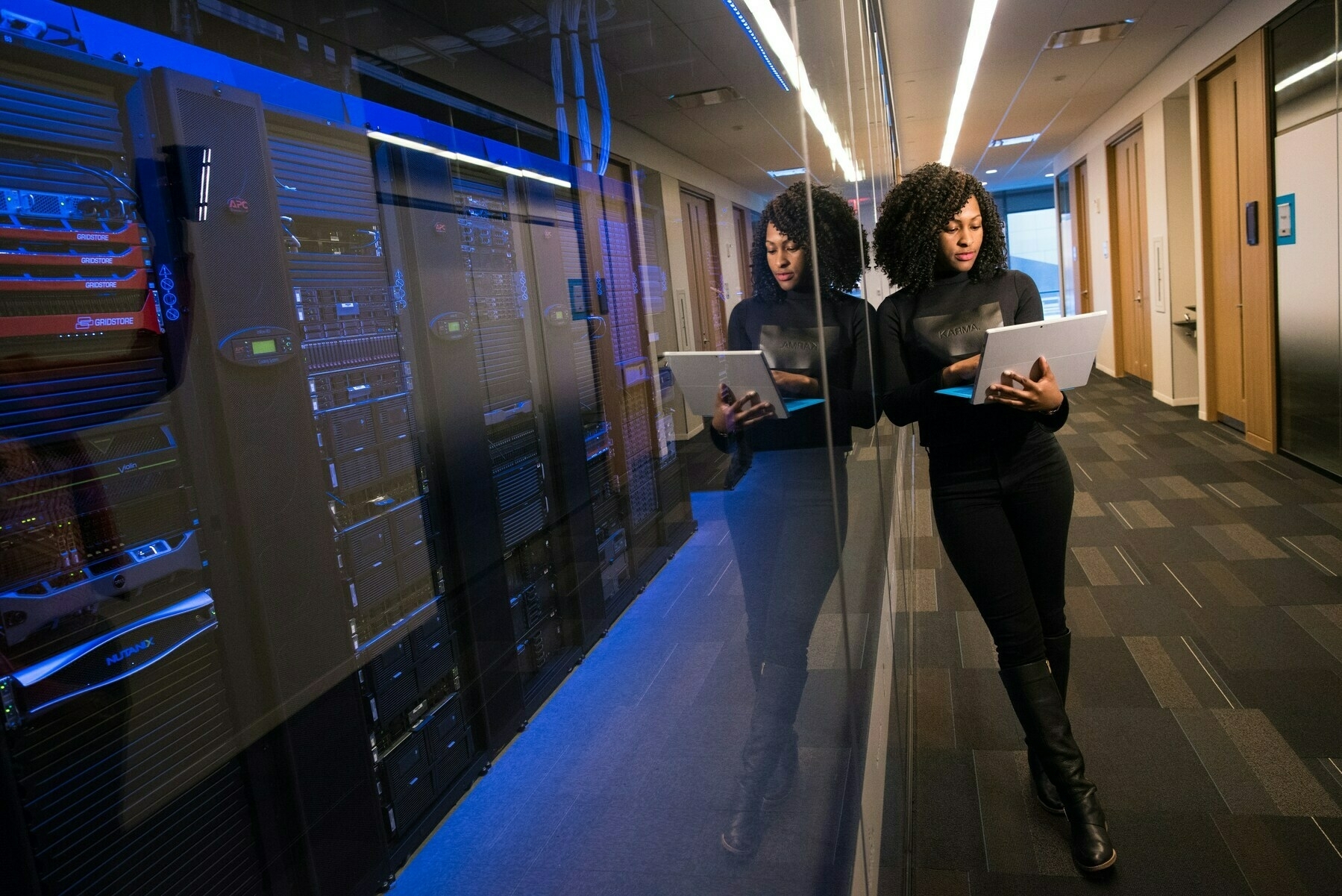
Should we even be using AI given the negative impact on climate, the environment, on disadvantaged people? Or, as Helgztech put it so delicately:
And you’re comfortable with exploiting the labor of those whose work was scraped to create the AI output? The unacknowledged writers and artists? And the underpaid workers who trained the models? And the power consumption?
Let’s recap that argument with a bit more detail. See citations at the end of this blog entry for links supporting each of Helgztech’s points.
Did You Know?
Major U.S. newspapers sue OpenAI, Microsoft for copyright infringement
Eight newspapers are suing OpenAI and Microsoft for using their articles to train AI systems without permission. The newspapers claim this is copyright infringement and want the AI systems to be stopped and destroyed. The lawsuit raises questions about copyright laws and their application to AI development. (AI generated summary of Axios article
The Problem with AI
If you’re wondering about Helztech’s assertions, let’s review them again:
- AI use exploits the labor of people in third world countries who have to sort through tons of yucky content (e.g. porn) to filter out what is appropriate and what is not. These are data labellers, delivery couriers, and content moderators who engage in large volumes of monotonous, mentally draining labor. Counter: Advocate for fair wages, better working conditions, and proper recognition for workers.
- AI steals from writers and artists, not giving them any credit for their work that it vacuumed up off the web and elsewhere to become a faceless, unattributable blog of information that it can draw upon when developing responses to the multitude of questions and prompts people ask it to respond to. Counter: In regards to data scraping and intellectual property, proper attribution, increased respect for IP rights, and compensation issues need to be worked out.
- AI uses inordinate amounts of power for hours to weeks during taining, causing equipment to run at high capacity. This results in increased energy consumption. AI equipment, due to its high capacity usage, results in the use of lots of energy, both for computation and for the liquid cooling systems (which involves a lot of water usage, often in places that can’t afford to spare the water) Counter: Ensure that AI system become more efficient and rely on clean energy sources going forward.
This can’t be the first time in human history technology has resulted in ethical challenges and issues.
Technology and Humanity: A Recap of Ethical Concerns
The following is sourced from Claude.ai. It suggests that many transformative technologies through history have dealt with similar ethical challenges: Here are some examples of other technologies in human history that faced similar ethical concerns, and how those issues were addressed:
Technology | Ethical Concerns | Resolutions |
---|---|---|
Industrialization and factories (late 18th to 19th century) | - Exploitation of labor, including child labor - Unsafe working conditions - Pollution and environmental damage |
- Labor laws and regulations (e.g., minimum wage, maximum work hours, safety standards) - Unionization and collective bargaining - Environmental regulations and policies |
Automobiles (early 20th century) | - Safety risks to drivers and pedestrians - Pollution and environmental impact - Displacement of workers (e.g., horse-drawn transportation) |
- Development of safety features (e.g., seat belts, airbags, crash testing) - Emissions standards and regulations - Transition to new jobs in the automotive industry |
Nuclear energy (mid-20th century) | - Safety risks and potential for accidents - Environmental impact of nuclear waste - Proliferation of nuclear weapons |
- Strict safety protocols and regulations - Development of safer reactor designs - International treaties and monitoring to prevent weapons proliferation |
Genetic engineering and biotech (late 20th century to present) | - Unintended ecological consequences - Ethical concerns around modifying life - Inequitable access and potential for enhancement |
- Regulations and oversight by agencies like the FDA and EPA - Bioethics committees and guidelines - Ongoing public dialogue and policy discussions |
Social media and online platforms (early 21st century to present) | - Privacy concerns and data exploitation - Spread of misinformation and echo chambers - Displacement of traditional media and journalists |
- GDPR and other data privacy regulations - Fact-checking and content moderation efforts - Antitrust scrutiny and discussions around regulation of tech giants |
I am sure that the table shown above doesn’t even come close to capturing the exploitation of labor, as well. As I write this, an example comes to mind. One that I grew up with as a boy in the Republic of Panama.
The Panama Canal
I grew up in the shadow of the Panama Canal, where an estimated total of 27,609 workers (many brought over from Caribbean countries) died during its construction. Those deaths came about as a result of yellow fever, malaria, and other tropical diseases.
However, the human toll doesn’t include the environmental impact, water usage, economic and social inequality of the Panamanian people, and Panamanian sovereignty.
Even if you consider all that’s wrong with the Panama Canal, its use continues today. The Panamanian government gets an average of $2.5 to $3.4 billion dollars from 2017-2021. Revenue generated by those who ship products through the Canal is in the billions.
Where there is money to be made, it’s the practice of human beings to do whatever it takes to get it. Like the Panama Canal, I don’t see human beings stopping all development and use of AI.
Al in Schools
Some would argue that these points do not negate the benefits and positive use cases of AI once the concerns are addressed. There’s nothing wrong with AI usage by the public since the money makers and everyone else already recognize the value of AI development and use.
You may be asking, “What about AI in schools?” Should we be using AI in schools given the valid points Helgztech makes? It’s really an ethical question, right? But it doesn’t matter at this point. We keep repeating a pattern of technology being pushed into schools, regardless of the ethical considerations.
In each case, there were edtech advocates who encouraged the use of technology in K-12 classrooms, modeling their use, staging learning events around it. If those people had, en masse, decided to do something else, what would have been the result? Would the technology still have found its way into schools?
In considering the examples that appear further down below, I asked Claude.ai those questions. I don’t disagree with the response:
If the edtech advocates (K-12 educators) had collectively decided not to promote or model the use of these technologies with ethical concerns, it’s likely that the adoption of these technologies in schools would have been significantly slower or more limited. Educators play a crucial role in shaping the use of technology in classrooms, and their endorsement or resistance can greatly influence the widespread adoption of any given technology.
In the absence of educator support, several scenarios could have unfolded:
Limited adoption: Without the advocacy and modeling by educators, many schools may have been less inclined to invest in or implement these technologies. This could have resulted in a more piecemeal or sporadic adoption, with only a few schools or districts choosing to use them.
Delayed implementation: The lack of educator buy-in could have delayed the widespread implementation of these technologies, giving more time for ethical concerns to be addressed or for alternative solutions to be developed.
Increased scrutiny: If educators had collectively raised concerns about the ethical implications of these technologies, it could have led to increased public scrutiny and pressure on the companies developing them. This could have prompted more proactive efforts to address the ethical issues before widespread adoption.
Alternative solutions: In some cases, the absence of educator support for a particular technology could have created a void that encouraged the development and adoption of alternative, more ethically-sound solutions.
However, it’s important to note that while educator support is a significant factor, it’s not the only one that influences the adoption of technology in schools. Other factors, such as market forces, political pressures, and the influence of technology companies, also play a role. In some cases, these factors may have still driven the adoption of certain technologies, even without the full support of educators.
Additionally, not all educators may have been aware of or concerned about the ethical implications of these technologies, and some may have advocated for their use despite these concerns.
In conclusion, while the collective decision of edtech advocates to not promote or model the use of ethically concerning technologies would have likely slowed or limited their adoption in schools, it may not have completely prevented their eventual implementation. Nonetheless, educators have a significant influence and responsibility in shaping the use of technology in K-12 classrooms, and their stance on ethical issues can make a substantial difference.
I’m not sure I could have said it better myself.
Examples of Technologies in K-12: 1980s and Onward
Both tables below were generated by Claude.ai:
Technology | Ethical Concerns | Resolutions |
---|---|---|
Channel One News (1990s) | - Commercialization of education - Forced exposure to advertising - Biased or inappropriate content |
- Criticism led some schools to opt-out or seek alternatives - Increased media literacy education efforts |
Internet access in schools (1990s) | - Exposure to inappropriate content - Online safety and privacy risks - Digital divide and unequal access |
- Implementation of content filters and firewalls - Digital literacy and cyber safety education - E-rate funding and initiatives to bridge the digital divide |
Webcams in classrooms (2000s) | - Privacy concerns for students and teachers - Potential for misuse or unauthorized access - Chilling effect on classroom behavior |
- Strict guidelines for webcam use and placement - Secure storage and access controls for recordings - Opt-out policies for students and teachers |
Biometric identification (2000s) | - Collection and storage of sensitive biometric data - Potential for data breaches and misuse - Consent and privacy concerns for minors |
- Strict data protection and encryption measures - Limit use to specific purposes (e.g., lunch lines) - Opt-out options and alternative identification methods |
RFID tracking (2000s) | - Surveillance and privacy concerns - Potential for data misuse or unauthorized access - Consent and privacy issues for minors |
- Limited use to specific purposes (e.g., library books) - Secure data storage and access controls - Opt-out options and alternative tracking methods |
Classroom management software (2010s) | - Excessive surveillance and control - Data privacy and security risks - Potential for misuse or bias in application |
- Transparent policies on data collection and use - Strict data protection measures and access controls - Regular audits and reviews for fairness and effectiveness |
Consider this list:
Here are some examples of technology being deployed in K-12 schools, along with their effectiveness, ethical concerns, and resolutions:
Technology | Deployment | Effectiveness | Ethical Concerns | Resolutions |
---|---|---|---|---|
Facial recognition systems | Used for attendance tracking, campus security, and monitoring student behavior | Mixed results; accuracy concerns, especially for students of color | Privacy violations, potential for misuse and bias, lack of student consent | Strict guidelines for use, opt-out policies, transparency about data collection and usage |
Online learning platforms (during COVID-19) | Widespread adoption for remote learning during school closures | Varied effectiveness; issues with access, engagement, and learning outcomes | Digital divide and inequity, student data privacy, screen time concerns | Providing devices and internet access, training for teachers and students, data protection policies |
AI-powered adaptive learning software | Personalized learning paths and content based on student performance | Promising results for individualized learning, but limited evidence of long-term efficacy | Algorithmic bias, privacy concerns, reduced teacher autonomy | Ensuring diverse training data, human oversight, and transparency; protecting student data; empowering teachers |
Social media monitoring tools | Tracking students' social media activity to identify potential threats or concerning behavior | Questionable effectiveness; high rates of false positives and limited prevention | Invasion of student privacy, chilling effect on free speech, disproportionate impact on marginalized students | Clear policies on monitoring scope, student and parent notification, opt-out options, data retention limits |
Virtual and augmented reality (VR/AR) | Immersive educational experiences and simulations | Engaging for students, but limited research on learning outcomes; high costs and technical challenges | Potential for physical discomfort, psychological impact, and addiction; unequal access | Age-appropriate content, time limits, adult supervision, ensuring equal access for all students |
Wearable devices (e.g., fitness trackers) | Tracking student physical activity and health data for PE classes and wellness programs | Can encourage physical activity, but accuracy and long-term engagement are concerns | Student privacy, data security, potential for body shaming or unhealthy competition | Opt-in policies, strict data protection, focusing on overall wellness rather than individual metrics |
Citations for Assertions
To support the assertions regarding problems with AI, consider the following:
Assertion | Supporting Citations |
---|---|
AI is exploiting the labor of those whose work was scraped to create the AI output. | [1], [2], [4], [9], [12] |
The unacknowledged writers and artists. | [4], [9], [15] |
The underpaid workers who trained the models. | [1], [2], [3], [13], [16], [17], [18] |
AI power consumption. | [3], [5], [6], [7], [8] |
Citations:
- [1] www.linkedin.com/pulse/art…
- [2] www.noemamag.com/the-explo…
- [3] www.spglobal.com/commodity…
- [4] www.aje.com/arc/ai-an…
- [5] www.scientificamerican.com/article/t…
- [6] www.vox.com/climate/2…
- [7] spectrum.ieee.org/ai-energy…
- [8] www.nytimes.com/2023/10/1…
- [9] www.creativindie.com/the-creat…
- [10] link.springer.com/article/1…
- [11] www.ll.mit.edu/news/ai-m…
- [12] hasdata.com/blog/web-…
- [13] theconversation.com/long-hour…
- [14] www.imf.org/en/Blogs/…
- [15] houstonlawreview.org/article/9…
- [16] www.washingtonpost.com/world/202…
- [17] https://www.reddit.com/r/technology/comments/zx6jm5/how_ai_innovation_is_powered_by_underpaid_workers/
- [18] www.bloomberg.com/news/arti…
- [19] www.axios.com/2024/04/0…